Faster R-CNN
This is an effort to improve R-CNN and Fast-CNN. The main improvements are :
-
The author uses a Region Proposal Network (RPN) that proposes boxes out of a given feature map which allows end-to-end training (previous methods used a third party proposal method).
-
They use multi-resolution anchor boxes
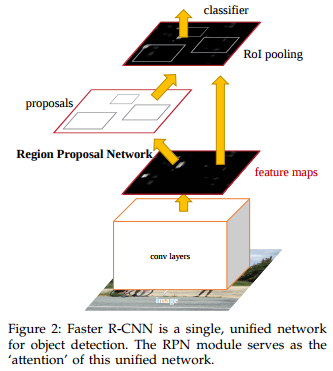
Conv layers
They use VGG-16 and the feature maps fed to the RPN are those in layer 5.
Region Proposal Network
The RPN shares the same features maps than the Faster RCNN network to allow end-to-end training. To generate region proposals, they slide a small network over the convolutional feature map returned by the last shared convolutional layer (in their implementation, layer 5 of VGG-16). This small network takes as input an \(n\times n\) spatial window of the input convolutional feature map and output a box-regression layer (reg) and a box-classification layer (cls) (c.f.Figure 3). This architecture is implemented with an \(n\times n\) convolutional layer followed by two sibling $1 \times 1$ convolutional layers
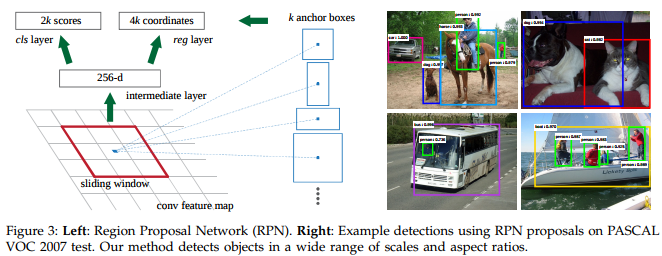
Loss function
The loss function is the sum of a cross-entropy for the labels and a regression loss between the predicted bounding box and the groundtruth boxes (1 box = 4 coordinates).
Results
FasterRNN gets respectively a mean AveragePrecision (mAP) of 78.8 and 75.9 on PASCAL VOC 2007 and 2012.
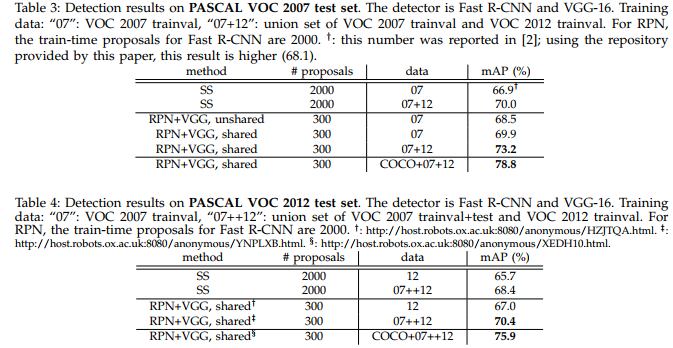
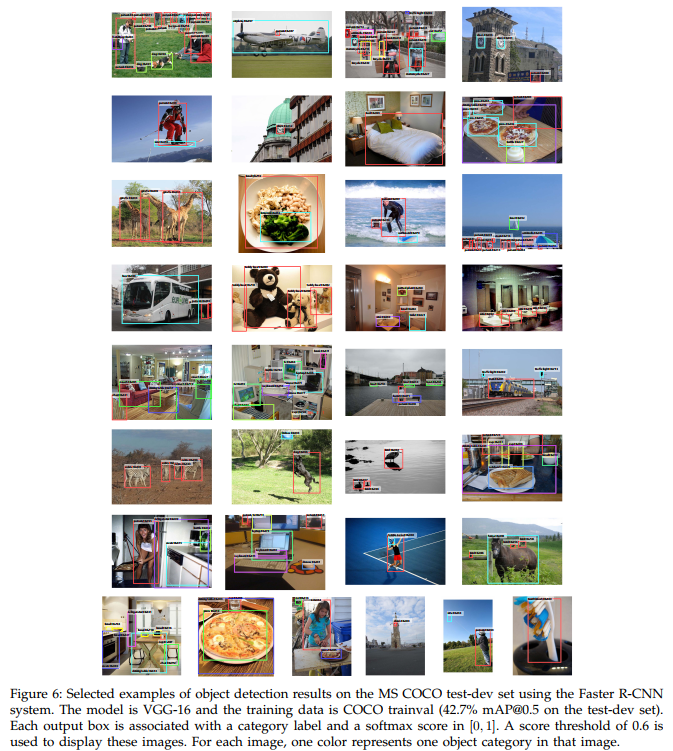