DelugeNet : Deep Networks with Massive and Flexible Cross-layer Information Inflows
DelugeNet is some sort of DenseNet but with a series of blocks
and composite layers
. The layers operating on the same feature map dimensions can be grouped to form a block
. The layers inside the block are fully connected as for DenseNet.
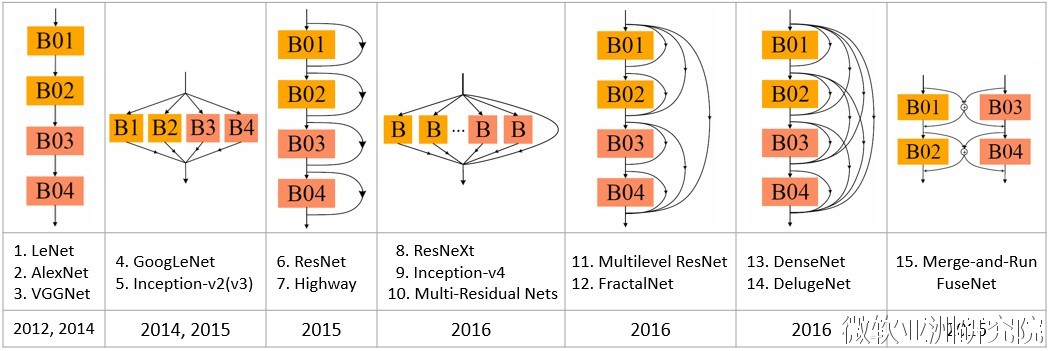
The layers inside a block are bottleneck
layers similar to that used in ResNet (c.f Fig.1(a)). The configuration of a block
is shown in Fig.1(c).
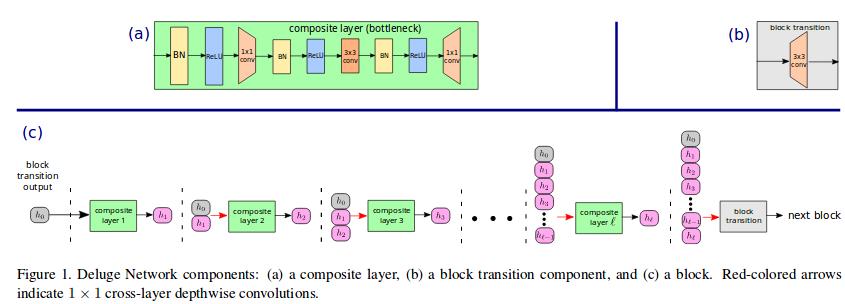
In order to reduce the number of parameters, DelugeNet uses before the bottleneck layer a cross-layer depthwise convolutional
layer (the red arrow in Fig.1) which concatenates the channels of feature map outputs of many layers, and then applies (channel,spatial)-independent filters to the concatenated channels (c.f. Fig.2). At the end of a block
is a transition
layer made of a cross-layer depthwise convolutional
layer followed by 3×3 spatial convolutional layer.
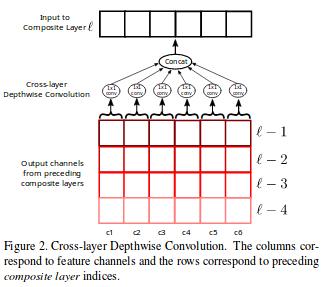
Results
Deluge net is slighly more accurate than Densenet on CIFAR100 (not really on CIFAR10 though) while having fewer parameters.
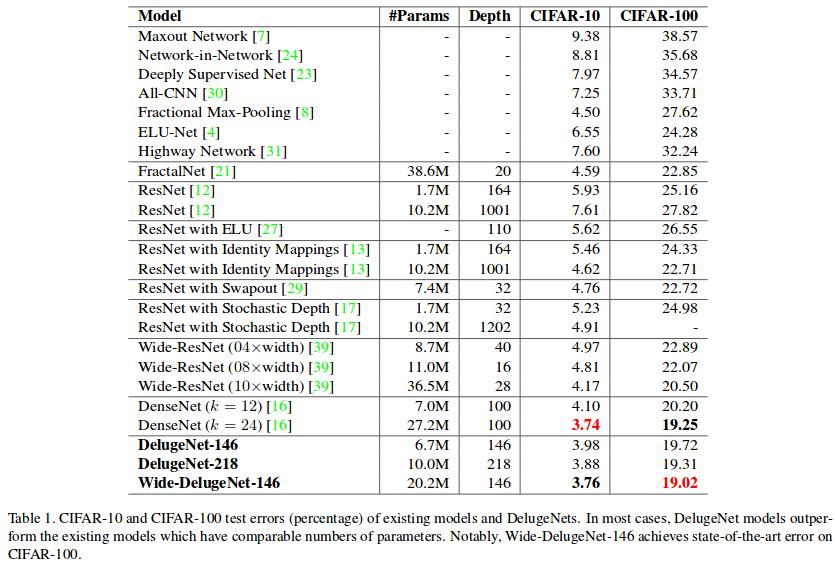